Beginners Guide to Paywall A/B Testing: Examples and Experiment Ideas You Can Try Today
Beginners Guide to Paywall A/B Testing: Examples and Experiment Ideas You Can Try Today

Tatev

Tatev
Jun 4, 2024
Jun 4, 2024
Developing an app is one thing but monetizing it is a whole other story. Even though you can build a great app with lots of useful features, it’s safe to say that app monetization strategies determine the success of your app, and hence, your revenue.
Among various app monetization strategies, paywalls are powerful tools for subscription-based apps to convert free users into paying customers. However, not all paywalls convert trial or freemium users effectively. Finding the optimal paywall setup for your audience is challenging, and this is where A/B testing becomes invaluable.
Why do you need in-app experiments, and what are effective A/B testing objectives? This article covers the basics of paywall A/B tests, identifying essential metrics, and formulating testing hypotheses so you have a clear understanding of how to work with Paywall Experiments in the future.
Developing an app is one thing but monetizing it is a whole other story. Even though you can build a great app with lots of useful features, it’s safe to say that app monetization strategies determine the success of your app, and hence, your revenue.
Among various app monetization strategies, paywalls are powerful tools for subscription-based apps to convert free users into paying customers. However, not all paywalls convert trial or freemium users effectively. Finding the optimal paywall setup for your audience is challenging, and this is where A/B testing becomes invaluable.
Why do you need in-app experiments, and what are effective A/B testing objectives? This article covers the basics of paywall A/B tests, identifying essential metrics, and formulating testing hypotheses so you have a clear understanding of how to work with Paywall Experiments in the future.
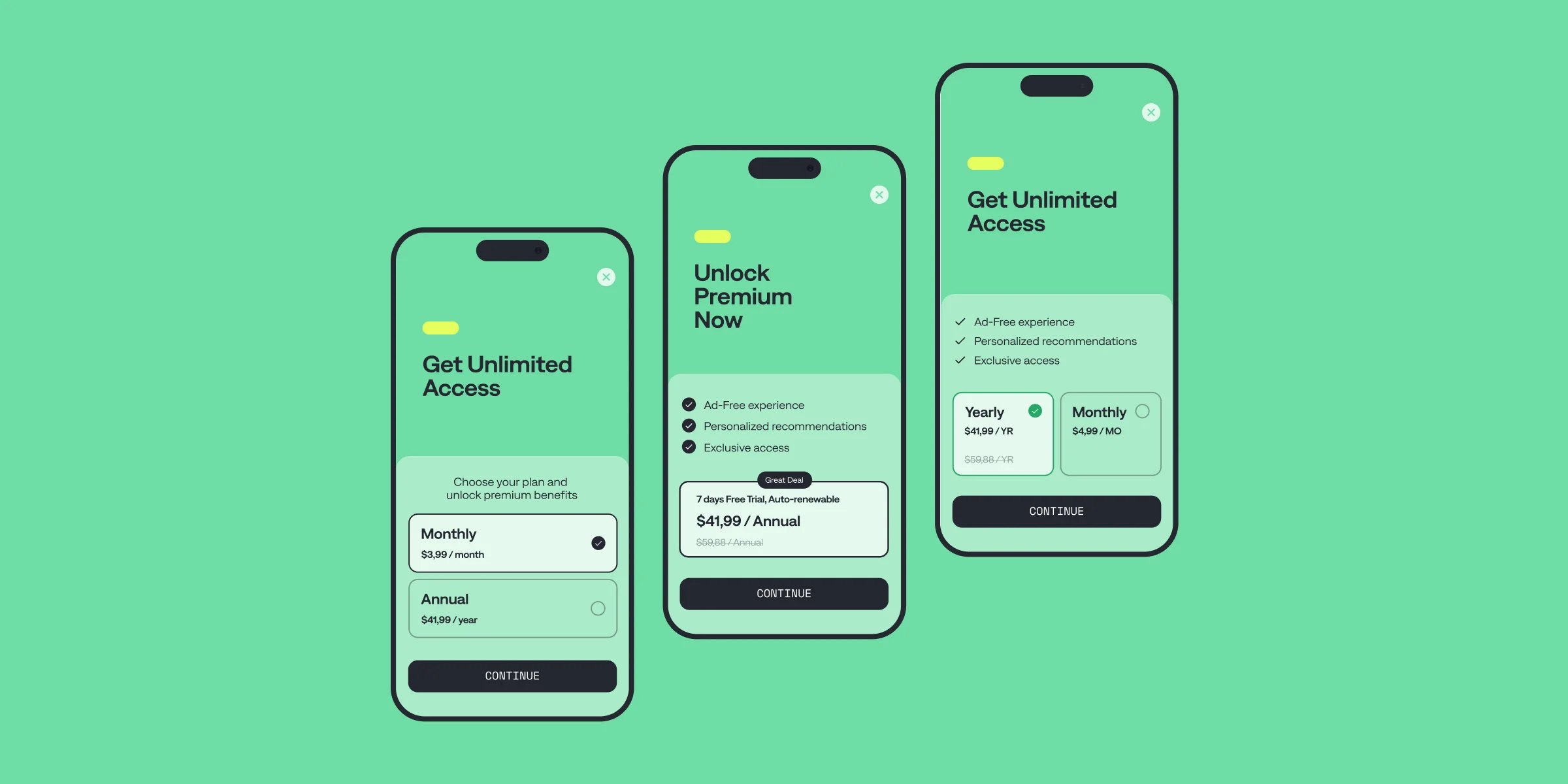
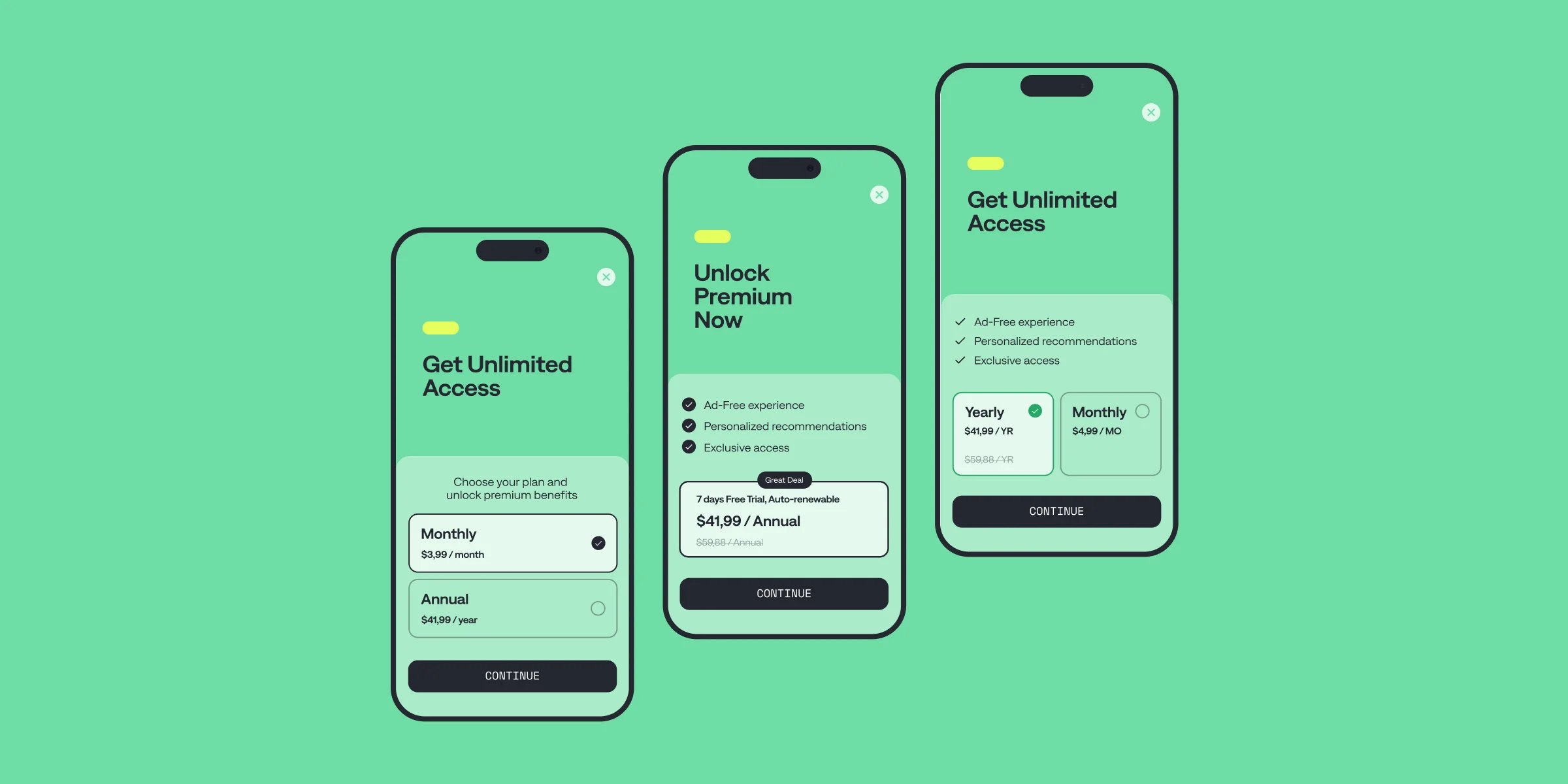
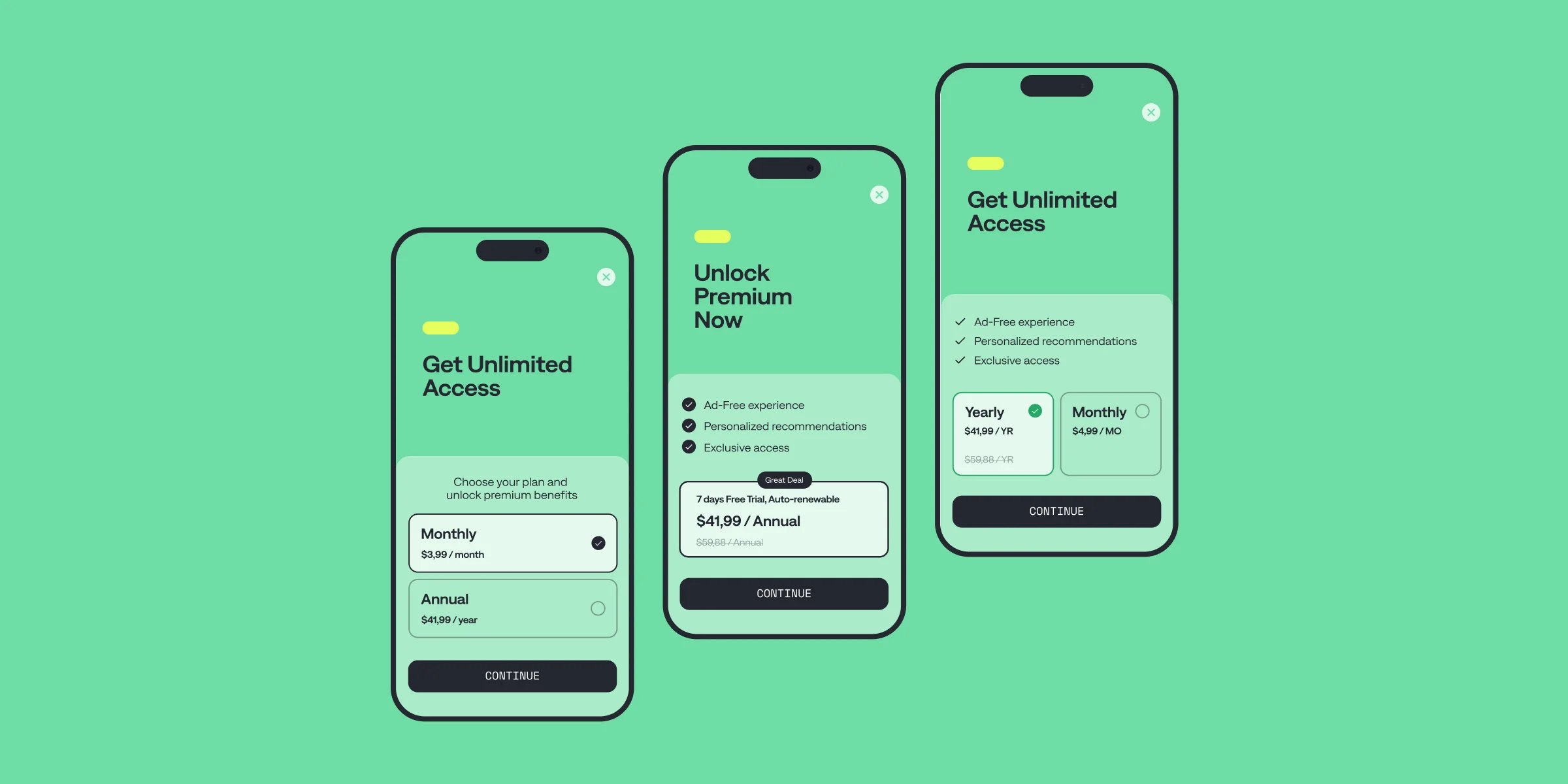
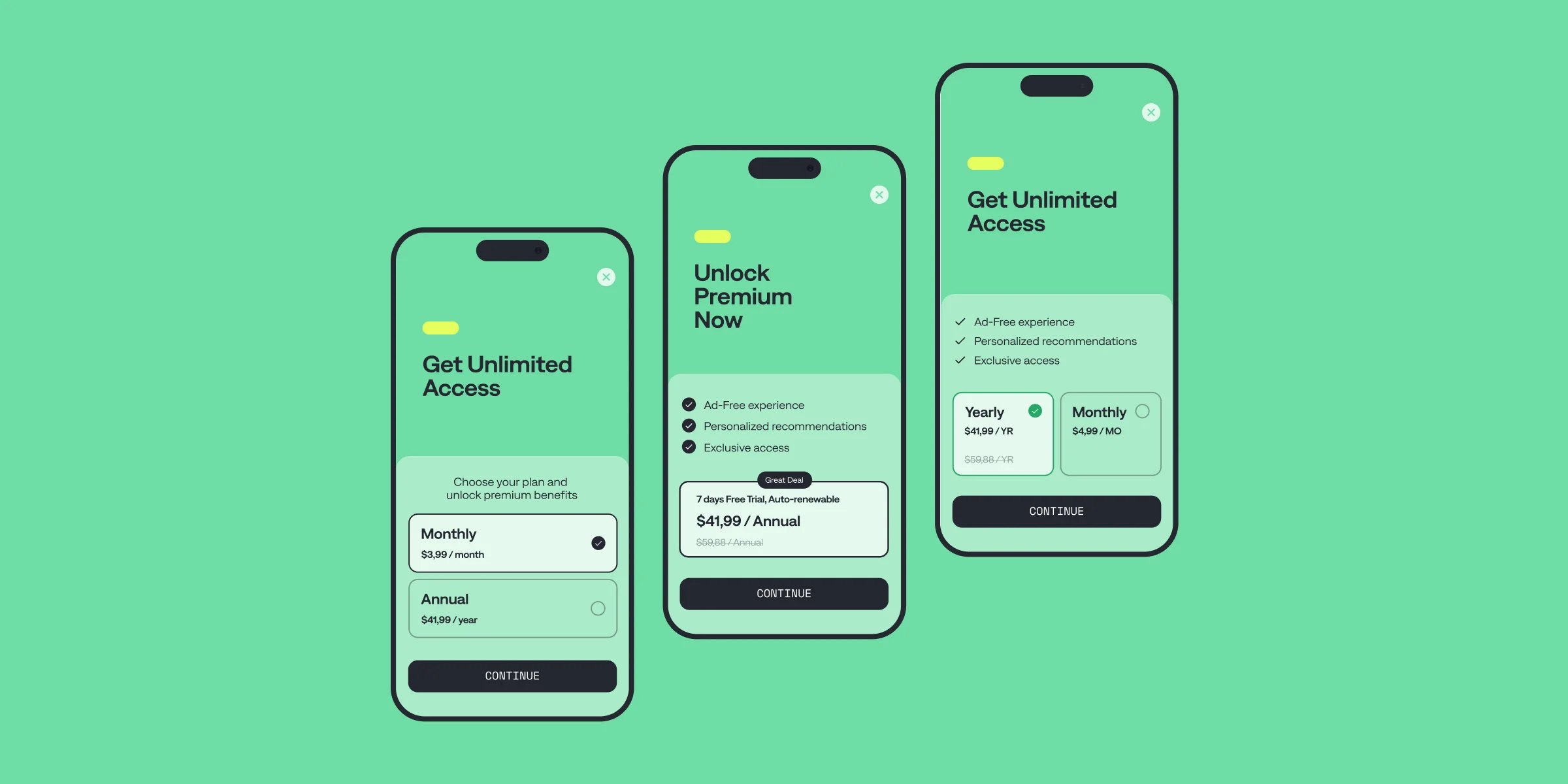